The alternative approach to patients’ randomization
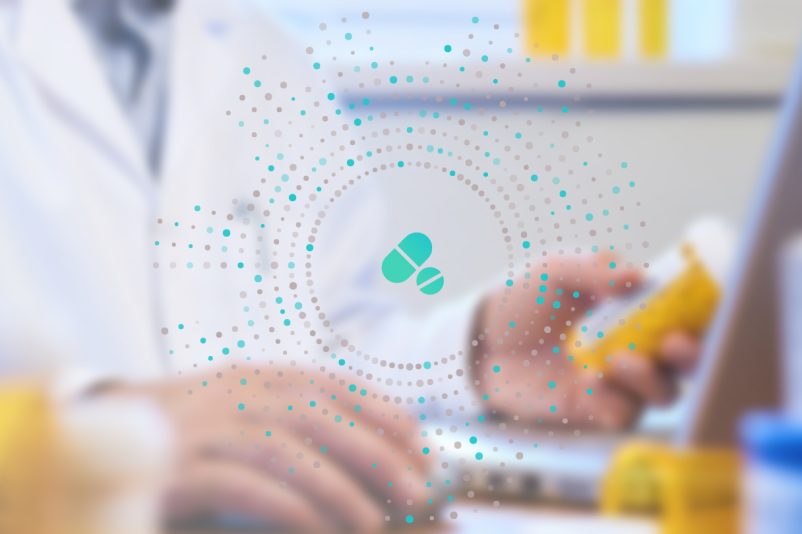
The demand for more randomized clinical trials increases continuously. Clinical researchers can control for bias in clinical trials with the help of randomization. It essentially eliminates the bias associated with treatment selection. Even if the concept of randomization looks simple, there are some very important issues related to balancing sample sizes and controlling the influence of covariates. Organizations often face challenges in achieving balanced randomization of patients through different groups. These issues appear mostly due to a randomization method, which is not suitable for the specific sample size or study needs and requirements. Various techniques have been implemented to address the need for balanced randomization of patients, including block, stratified randomization, and covariate adaptive techniques. They all come with their pros and cons, and they are all suitable in certain situations and irrelevant in others.
Clinicubes provides flexible randomization capabilities, which self-adapt to the study needs, no matter the expected number of subjects or how many covariates will be controlled. The Clinicubes randomization tool takes into account present group sizes and patient characteristics in each group and automatically adapts the randomization method for each patient upon his assignment. In addition, Clinicubes allows its users to set or change randomization methods according to clinical trials’ needs.
However, before we dive deeper into its capabilities let’s take a quick look at available randomization methods.
Simple Randomization
Let’s first review simple randomization – flipping the coin. The method allows fully randomized enrollments and can be easily applied in clinical trials. Still, a potential problem can be observed with small clinical trials, with a population sample smaller than 100. In such trials, the simple randomization methods may result in imbalanced sample size among the control and treatment groups.
Block Randomization
Then we look at the block randomization. This method is suitable for small populations and ensures balance in the group sizes. However, the generated groups might be hardly comparable, due to imbalance in the patients’ characteristics (covariates) which are important for the trial results. For example, one group may have more participants with concomitant diseases or other prerequisites that can compromise the data and potentially influence the results of the clinical trial. Such an imbalance can lead to deviations in statistical analysis and reduce the strength of the survey. Therefore, sample size and covariates should be balanced in clinical trials. It is possible to lose balance in the individual groups in terms of criteria important for the study, for example, in one of the groups we may get more patients with concomitant diseases. This leads to a subsequent deviation in statistical analysis and serious concerns in the interpretation of the survey results. These concerns are exacerbated by the size of the study itself. The smaller the population, the more complex a model is needed.
Stratified Randomization
The stratified method of randomization focuses on the need to control and balance the influence of covariates. This method is based on the theory of probability and can achieve a balance between groups considering the main characteristics of the patients in the population. Stratified randomization provides a separate block for each combination of patients’ characteristics. Each of these patients is assigned to the respective block, and after all participants are been assigned, simple randomization is done within each block. Although stratified randomization is a relatively simple and useful technique, especially for smaller clinical trials, it becomes complicated to implement if many covariates must be controlled. The model has another limitation as well – it works only when all subjects are known before the groups’ assignment. However, this method is rarely applicable because clinical research subjects are often enrolled one at a time on a continuous basis. In such cases, an alternative approach for patient randomization is needed.
Covariate Adaptive Randomization
Many researchers have recommended covariate adaptive randomization as a valid alternative randomization method for clinical research. It allows organizations to start with a small sample population, which will grow with time. The method takes into account the characteristics of previously assigned patients and assigns new patients according to present distribution through groups. Covariate adaptive randomization uses the method of minimization by assessing the imbalance of sample size among several covariates.
Clinicubes’ Randomization model
Clinicubes’ randomization tool is based on the covariate adaptive randomization method but enhances its advantages with self-adaptation capabilities ensuring the balance in the different groups. As mentioned above in the clinical trials patient are often enrolled one by one. In this scenario, we go through different sample sizes and those sizes need to be taken into account. Clinicubes’ randomization tool eliminates those challenges with a combination of randomization methods and automatically adapts the randomization method regardless of the sample size. Another key advantage of the randomization tool is that is fully unpredictable. Unlike other randomization models, with Clinicubes you always will receive different results no matter how many times you do the same randomization simulation.
Bellow is illustrated how 1260 patients can be randomized with Clinicubes in 3 groups.
- Randomized Patients TOTAL for Group A: 420
- Randomized Patients TOTAL for Group B: 420
- Randomized Patients TOTAL for Group C: 420
Observation matrix – All randomized patients at the moment:
Rules\Arms | Group A | Group B | Group C |
Blood Pressure – 180-200 | 224 | 225 | 223 |
Blood Pressure – 160-180 | 196 | 195 | 197 |
BMI – Obesity | 146 | 145 | 144 |
BMI – Overweight | 112 | 113 | 113 |
BMI – Underweight | 49 | 49 | 50 |
BMI – Normal Weight | 113 | 113 | 113 |
Concomitant diseases – present | 216 | 216 | 217 |
Concomitant diseases – not present | 204 | 204 | 203 |
Height – 180-195 | 103 | 102 | 104 |
Height – 165-180 | 154 | 153 | 151 |
Height – 150-165 | 119 | 120 | 121 |
Height – 195-200 | 44 | 45 | 44 |
Sex – Male | 213 | 211 | 212 |
Sex – Female | 207 | 209 | 208 |
Smoker – Yes | 215 | 216 | 213 |
Smoker – No | 205 | 204 | 207 |
The required balance is achieved.
The advantages of Clinicubes CTMS don’t stop here. It provides various benefits and integrated solutions for every aspect and phase of the clinical studies. This clinical trial management system is highly focused on budgeting, finance tracking, and reporting. The system accommodates the needs of research organizations to collect, retain, document, and store scientific and patient data. Furthermore, it tracks deadlines, schedules visitations as well as monitors the whole progress resulting in better control of all activities, better team collaboration, and up-to-date access to all required data.
Get in touch and take advantage of what our clinical trial management system offers.